À lire dans le dernier numéro de Wired sur l’IA | How to Teach Artificial Intelligence Some Common Sense
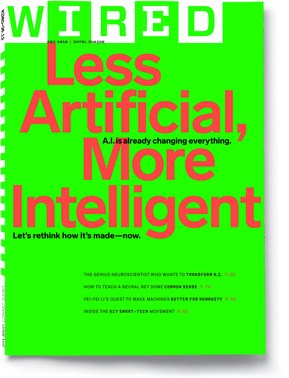
Five years ago, the coders at DeepMind, a London-based artificial intelligence company, watched excitedly as an AI taught itself to play a classic arcade game. They’d used the hot technique of the day, deep learning, on a seemingly whimsical task: mastering Breakout,1 the Atari game in which you bounce a ball at a wall of bricks, trying to make each one vanish.
Deep learning is self-education for machines; you feed an AI huge amounts of data, and eventually it begins to discern patterns all by itself. In this case, the data was the activity on the screen—blocky pixels representing the bricks, the ball, and the player’s paddle. The DeepMind AI, a so-called neural network made up of layered algorithms, wasn’t programmed with any knowledge about how Breakout works, its rules, its goals, or even how to play it. The coders just let the neural net examine the results of each action, each bounce of the ball. Where would it lead?
To some very impressive skills, it turns out. During the first few games, the AI flailed around. But after playing a few hundred times, it had begun accurately bouncing the ball. By the 600th game, the neural net was using a more expert move employed by human Breakout players, chipping through an entire column of bricks and setting the ball bouncing merrily along the top of the wall.
“That was a big surprise for us,” Demis Hassabis, CEO of DeepMind, said at the time. “The strategy completely emerged from the underlying system.” The AI had shown itself capable of what seemed to be an unusually subtle piece of humanlike thinking, a grasping of the inherent concepts behind Breakout. Because neural nets loosely mirror the structure of the human brain, the theory was that they should mimic, in some respects, our own style of cognition. This moment seemed to serve as proof that the theory was right.
…
Ce contenu a été mis à jour le 29 novembre 2018 à 16 h 54 min.